基于数字水印的深度神经网络版权保护技术研究
来源:56doc.com 资料编号:5D21020 资料等级:★★★★★ %E8%B5%84%E6%96%99%E7%BC%96%E5%8F%B7%EF%BC%9A5D21020
资料以网页介绍的为准,下载后不会有水印.资料仅供学习参考之用. 密 保 惠 帮助
资料介绍
基于数字水印的深度神经网络版权保护技术研究(论文15000字)
摘要:深度神经网络训练模型的共享是近年来深度学习系统研究和开发的一个重要方面,而训练一个优秀神经网络需要耗费大量人力物力,因此,深度神经网络训练模型的版权保护具有重要意义。为此,本文决定使用数字水印技术来保护训练模型的版权,并通过训练过的模型来检测侵犯训练模型版权的行为。
本文分析了在深度神经网络中嵌入数字水印的嵌入目标、嵌入情况、嵌入需求以及可能的攻击类型。最后,通过全面的实验证明了基于数字水印的深度神经网络版权保护算法的有效性,并且揭示了数字水印在保护深度神经网络模型版权这项研究的潜力。
关键词:数字水印;深度神经网络;残差网络
Research on Copyright Protection of Deep Neural Network Based on Digital Watermarking
Abstract:In recent years,the sharing of the deep neural network model sharing is an important aspect of deep learning system research and development, whereas the training of the model will expand a lot of time and money.Therefore, it is necessary to protect the copyright of deep neural network training model.This paper decides to use digital watermarking technology to protect the copyright of the training model and detect the infringement of the copyright of the training model through the trained model.
This paper analyzes the embedded target, embedded situation, embedded requirement and possible attack types of embedded digital watermark in deep neural network.Finally, the effectiveness of the deep neural network copyright protection algorithm based on digital watermarking is proved through comprehensive experiments, and the potential of digital watermarking in copyright protection of deep neural network model is revealed.
Key words:Digital watermarking; Deep neural network; Residual network
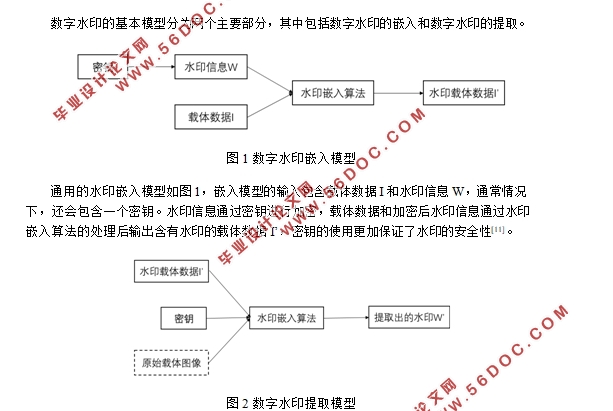
目录
1. 引言 1
1.1研究目的 1
1.2研究背景与研究意义 1
1.3本文的研究内容 1
1.4论文结构安排 1
2. 相关技术简介 2
2.1数字水印技术 2
2.1.1数字水印的基本概念 2
2.1.2数字水印的发展和现状 3
2.1.3数字水印的特性 4
2.1.4数字水印的性能评估标准 4
2.1.5数字水印的常见攻击类型 5
2.2深度神经网络 5
2.2.1人工神经网络 5
2.2.2深度神经网络 6
3. 基于数字水印的深度神经网络版权保护算法 7
3.1算法总体设计 7
3.1.1嵌入目标 8
3.1.2需求分析 8
3.1.3嵌入情况 9
3.1.4可能的攻击类型 10
3.2水印嵌入 10
3.2.1嵌入目标再明确 10
3.2.2数字水印的嵌入过程 11
3.3水印提取 13
3.4算法实现 13
3.4.1Keras介绍 13
3.4.2Numpy介绍 13
3.4.3正则化矩阵 14
3.4.4嵌入损失函数 14
4. 实验 15
4.1实验设置 15
4.1.1神经网络的选择 15
4.1.2训练参数设置 16
4.1.3数据集 16
4.2创建残差网络 17
4.3实验过程及结果分析 17
4.3.1训练嵌入 17
4.3.2微调嵌入 19
4.3.3数字水印的鲁棒性 19
4.3.4两个σ(x)函数的比较 20
5. 总结 21
5.1结论 21
5.2未来工作 21
5.2.1直接嵌入 21
5.2.2数字水印的鲁棒性研究 21
5.2.3其他加密方式 21
5.2.4水印覆盖 21
参考文献: 22
附录 24
致谢 28
|