电机轴承异常数据采集与分析方法(任务书,开题报告,外文翻译,论文18000字,实验报告,matlab主要程序)
摘要
轴承作为电机高速旋转运动摩擦转化的零部件,轴承的早期故障对电机的工作性能的影响也很大,特别是对于高精度制造装备来说,电机轴承的工作性能直接影响着设备的加工精度。现如今,对电机的状态监测和故障诊断的研究不再是利用故障发生后的信号分析,而是更多地关注故障形成的早期信号。
论文主要研究了电机轴承早期故障的诊断识别,建立了利用电机轴承早期故障信号的诊断网络。首先,了解电机初期故障识别的国内和国外的发展现状,以及现有的监测技术和故障评估技术。通过了解电机轴承的结构及其振动特性,分析其发生故障的机理,并简单介绍了轴承几种常见部位出现故障时,其对应的特征频率。
然后,根据分层抽样原则选取了实验数据,并借助数学计算分析软件Matlab和数字信号处理方法,对选取的轴承退化实验中采集到的振动(加速度)信号,按照分析要求和信号特点对其进行降噪滤波后,利用时域和频域内各种特征参数对轴承故障程度进行初步的判断。在分析过程中,提取了每个采样点的各类特征值,从而了解轴承的退化过程中,轴承的信号特征的变化,为特征量提供一定的依据。
接着,选取了合适的特征值作为轴承状态判定的依据。最后,在故障识别过程中通过神经网络对电机轴承的初期故障识别分类,并对不同特征参数的分类效果进行评估和对比。确定了最后的电机轴承的早期故障识别模型,实现了对电机轴承工作阶段的识别。
关键词:早期故障分析;电机轴承;MBVSA;神经网络模式识别
Abstract
Bearing as a part of friction transformation of high-speed rotating motion of motor, the early failure of bearing has a great impact on the working performance of motor, especially for high-precision manufacturing equipment, the working performance of motor bearing directly affects the machining accuracy of equipment.Nowadays, the research of motor condition monitoring and fault diagnosis is no longer using the signal analysis after the fault occurs, but paying more attention to the early signal formed by the fault.
This paper mainly studies the diagnosis and identification of early failure of motor bearing, and establishes a diagnosis network using early failure signal of motor bearing.Firstly, understand the domestic and foreign development status of motor initial fault identification, as well as the existing monitoring technology and fault assessment technology.Through understanding the structure and vibration characteristics of the motor bearing, the failure mechanism is analyzed, and the corresponding characteristic frequency of several common parts of the bearing is briefly introduced.
Then, according to the principle of stratified sampling to select the experimental data, and by means of mathematical analysis software Matlab and digital signal processing method, the selection of bearing degradation experiments, vibration (acceleration) signals were collected in accordance with the requirements for analysis and signal characteristics of noise filtering, using time domain and frequency domain feature parameters for preliminary judgment of the bearing fault degree.In the process of analysis, all kinds of characteristic values of each sampling point are extracted, so as to understand the change of bearing signal characteristics in the degradation process, and provide a certain basis for the characteristic quantity.
Then, the proper characteristic values are selected as the basis for determining the bearing state.Finally, in the process of fault identification, neural network is used to classify the initial faults of motor bearings, and the classification effects of different characteristic parameters are evaluated and compared.Finally, the early fault identification model of motor bearing is established, and the identification of motor bearing working stage is realized.
Key Words:Early failure analysis;Motorbearing;MBVSA;Neural network pattern recognition
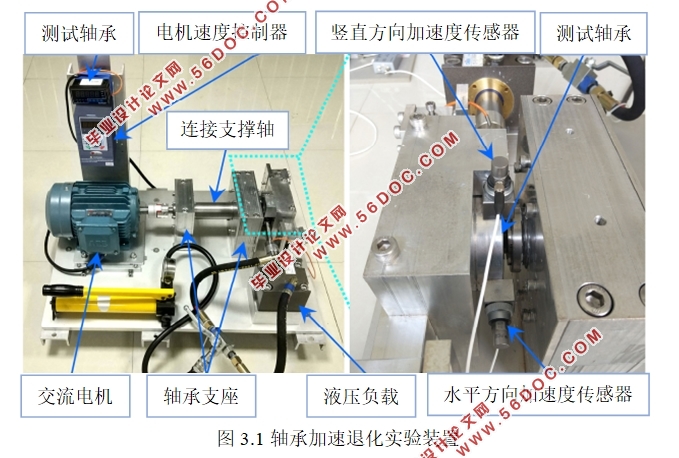
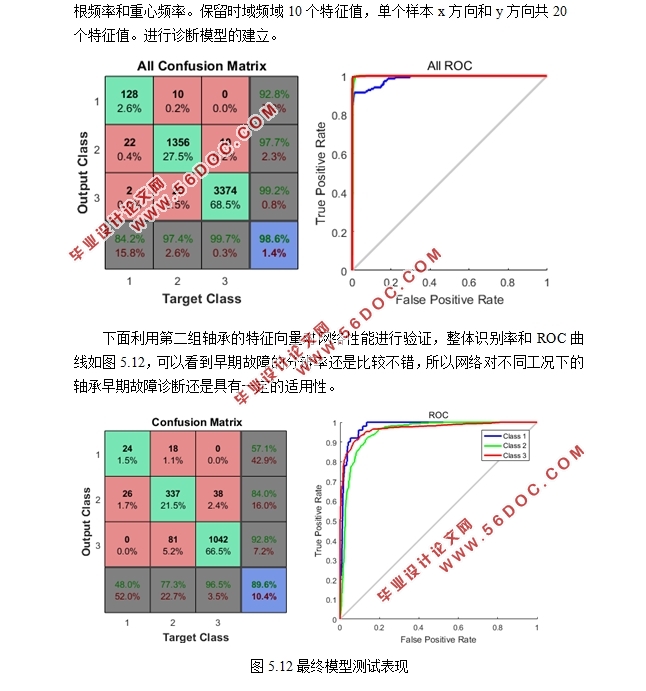
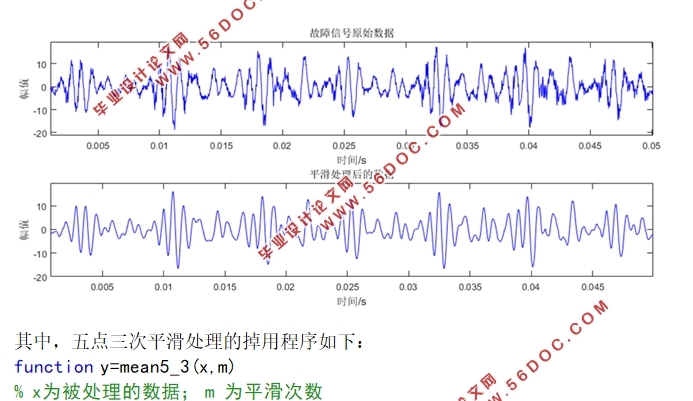
目录
第1章 绪论 1
1.1 研究背景及意义 1
1.2 国内外研究现状 2
1.3 现有振动信号分析方法 3
1.4 现有故障诊断技术 4
1.5 论文主要研究内容 5
第2章 电机轴承的结构及其振动机理 7
2.1 电机轴承的结构特征 7
2.2 电机轴承的故障机理及原因 7
2.2.1 电机轴承故障机理 7
2.2.2 电机轴承失效形式和原因 8
2.3 电机轴承的振动频率 9
2.3.1 轴承的固有频率 9
2.3.2 轴承的故障特征频率 10
第3章 电机轴承故障振动信号的采集 12
3.1 实验系统 12
3.1.1 实验系统结构及原理 12
3.1.2 被测轴承主要参数 13
3.2 振动数据的采集 13
3.2.1 样本数据采样参数 13
3.2.2 实验数据选取 14
第4章 振动信号的处理及分析方法 15
4.1 振动信号的预处理 15
4.1.1 消除趋势项 15
4.1.2 数据平滑处理 16
4.1.3 数字滤波器的选用 19
4.2 振动信号的时频域分析 21
4.2.1 振动信号时域分析 21
4.2.2 振动信号频域分析 22
4.3 信号时频域特征参数及分析 23
4.3.1 常见信号时频域特征参数 23
4.3.2 特征参数分类及特点 24
4.3.3 特征参数变化分析 25
第5章 人工神经网络轴承初期故障识别 27
5.1 神经网络原理及特点 27
5.1.1神经网络基础模型—人工神经元 27
5.1.2 神经网络的分类 28
5.2初期故障识别模型 29
5.2.1 特征值状态相关 29
5.2.2 神经网络的建立过程 30
5.2.3早期故障诊断网络训练 30
第6章 结论 34
6.1 全文总结 34
6.2 未来展望 34
参考文献 35
致 谢 37
|